Ryan Bahlous-Boldi
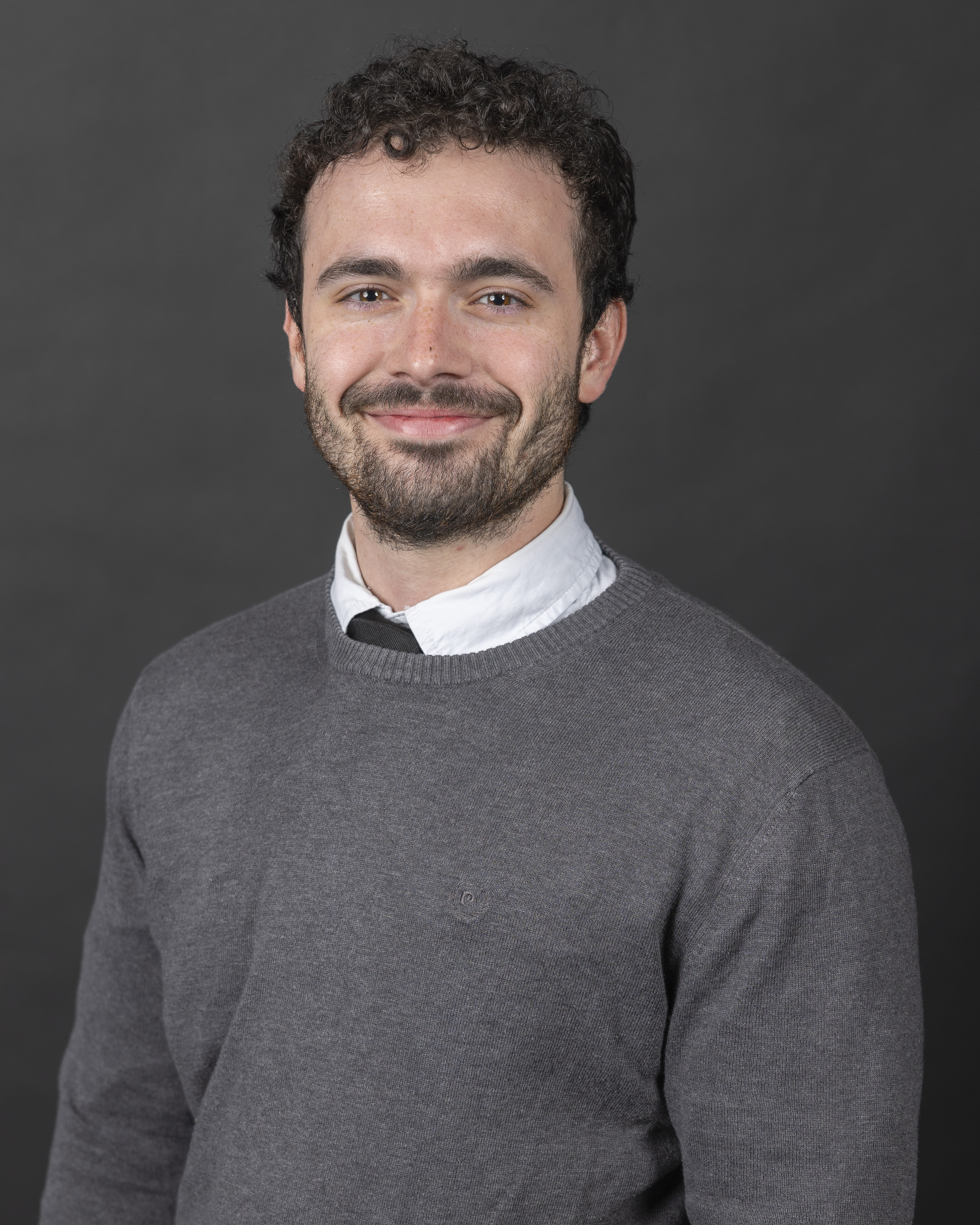
I’m an undergrad at UMass Amherst, researching how intelligence can emerge in adaptive artificial systems that evolve and learn. My focus is on enabling agents to adapt in dynamic, open-ended environments, understanding how intelligence emerges from simple rules, and designing systems that capture the complexity and generality of human intelligence.
On the other hand, I’m also interested in how we can leverage AI systems to help humans. I am interested in how we can model human preferences and behaviors to enable AI systems to be effective collaborators with humans.
With guidance from my mentors, I’m diving into key questions that drive my work:
- With Lee Spector, I’m exploring program synthesis with genetic programming. I design and optimize selection algorithms for multi-modal and multi-objective optimization.
- Working with Scott Niekum, I’m designing systems that infer reward functions in Reinforcement Learning from Human Feedback (RLHF) to help robotic and LLM agents better align to the diversity of human preferences.
- Alongside Stefanos Nikolaidis at USC, I leveraged Quality Diversity Optimization to improve adaptability in Reinforcement Learning for 3D robotic control tasks.
- With Katia Sycara at CMU, I investigated collaborative systems where LLM-based agents can work dynamically with human teams.
I am applying to PhD programs for Fall 2025. Through approaches like Genetic Programming, Program Synthesis, Reinforcement Learning, Robotics, and Cognitive Science, I hope to build systems that further our understanding of intelligence in autonomous agents and ultimiately in ourselves.
News
Dec 19, 2024 | I am excited to announce that I have been selected as a 2025 HRI Pioneer by the IEEE/ACM International Conference on Human-Robot Interaction. Looking forward to seeing you all in Melbourne! |
---|---|
Oct 28, 2024 | Some of our work on Pareto Optimal Preference Learning (POPL) was accepted to the Pluralistic Alignment workshop at NeurIPS 2024. |
Jun 3, 2024 | Exicted to be at Carnegie Mellon University’s Robotics Institute this summer working with Dr. Katia Sycara on emergent communication between diverse agents in multi-agent reinforcement learning settings. |
Mar 29, 2024 | Happy to announce that I was selected as a 2024 Goldwater Scholar! I am grateful for the support of my mentors, friends, and family. This year, 438 scholarships were awarded to undergrads in the US, with only 30 going to students in the field of Computer Science. |
Mar 10, 2024 | 3 short papers accepted to GECCO 2024! Amongst them is some work on integrating Quality Diversity Optimization with Reinforcement Learning, and an extension of our work on the interaction between selection and down-sampling training sets. I am excited to present this work in Melbourne, Australia this July! |
Representative Papers
-
Pareto-Optimal Learning from Preferences with Hidden ContextarXiv preprint arXiv:2406.15599 2024
-
Objectives Are All You Need: Solving Deceptive Problems Without Explicit Diversity MaintenanceIn The Workshop on Agent Learning in Open-Endedness (ALOE) at the Conference on Neural Information Processing Systems (NeurIPS) 2023
-